Key takeaways
- The Guide provides recommended best practices to mitigate the privacy risk of potential re-identification of synthetic data through governance controls, contractual processes and technical measures.
- The Guide will be useful to CIOs, CTOs, CDOs, data scientists, data protection practitioners and technical decision-makers involved in the generation and use of synthetic data.
- Businesses should carefully evaluate how any proposed use of synthetic data may impact data protection compliance overall, with a view to taking necessary steps to mitigate risks of re-identification and protect any personal data.
In more detail
Synthetic data generation is an increasingly popular privacy-enhancing technology based on data obfuscation, and it involves the generation of artificial data by a purpose-built mathematical model.
The PDPC recognises that while synthetic data is generally fictitious data that may not be considered personal data on its own, it is not inherently privacy risk-free due to possible re-identification risks.
Accordingly, the Guide seeks to recommend good practices, in the context of three common use cases, which organisations may adopt to reduce privacy risks when generating synthetic data.
These use cases and corresponding best practices are as follows:
- Use case: Generating training dataset for AI models, including data augmentation and increasing data diversity
Good practice: Adding noise in appropriate scenarios to, or reduce the granularity of, synthetic data points
- Use case: Data analysis and collaboration, including data sharing and analysis and previewing data for collaborative purposes
Good practice: Incorporating data protection measures through the synthetic data generation process, such as removing outliers from source data and pseudonymising source data during the data preparation phase
- Use case: Software testing, including system development to avoid data breaches
Good practice: Generating synthetic data that follow the semantics (e.g., format) of source data instead of statistical characteristics and properties; data protection measures through the synthetic data generation process, such as removing outliers from source data and pseudonymising source data during the data preparation phase
Additionally, PDPC recommends a set of good practices and risk assessments/considerations for generating synthetic data. These good practices and recommendations (found in Annex A of the Guide) are condensed into a five-step approach illustrating the synthetic data generation process, as summarised below:
- Step 1 – Know your data: Organisations should be clear on the purpose and use cases of the synthetic data and the source data that the synthetic data is to mimic. Organisations should establish objectives prior to synthetic data generation to determine an acceptable risk threshold of the generated synthetic data and expected utility of the data. Such acceptance criteria should be incorporated into the organisation's risk assessments or data protection impact assessment.
- Step 2 – Prepare your data: Organisations should consider: (a) the key insights that need to be preserved in the synthetic data; and (b) the necessary data attributes for the synthetic data to meet business objectives. Organisations should take note that the more closely the source data is mimicked, the greater the re-identification risk and the need for risk mitigation measures will be.
- Step 3 – Generate synthetic data: Organisations have to consider which method of synthetic data generation is most appropriate based on use cases, data objectives and data types. Thereafter, organisations should perform checks on the generated synthetic data to ascertain: (a) data integrity; (b) data fidelity; and (c) data utility. Before generating synthetic data, organisations may also consider splitting the source data into a training dataset and a control dataset for assisting re-identification risks.
- Step 4 – Assess re-identification risks: After the utility measurement of the generated synthetic data is found to be acceptable, organisations should assess and perform a re-identification risk assessment based on their internal acceptance criteria. Since the risk of re-identification cannot be deduced directly from examining whether the generated synthetic data contains any personal data, the re-identification risk assessment will be an evaluation based on re-identification attacks (e.g., singling out attacks, linkability attacks and inference attacks). There are various approaches to such risk assessment (e.g., disclosure approach, privacy risk threshold scores approach, and privacy integrity audit approach) that organisations can perform individually or engage a synthetic data solution provider to do so.
- Step 5 – Manage residual risks: Organisations should identify and mitigate all potential residual risks, including the potential impact on groups of individuals due to membership disclosure and model leakage. These risks and mitigation controls should be documented and approved by management and key stakeholders as part of the organisation's overall enterprise risk framework.
* * * * *
For further information and to discuss what this might mean for you, please get in touch with your usual Baker McKenzie contact.
* * * * *
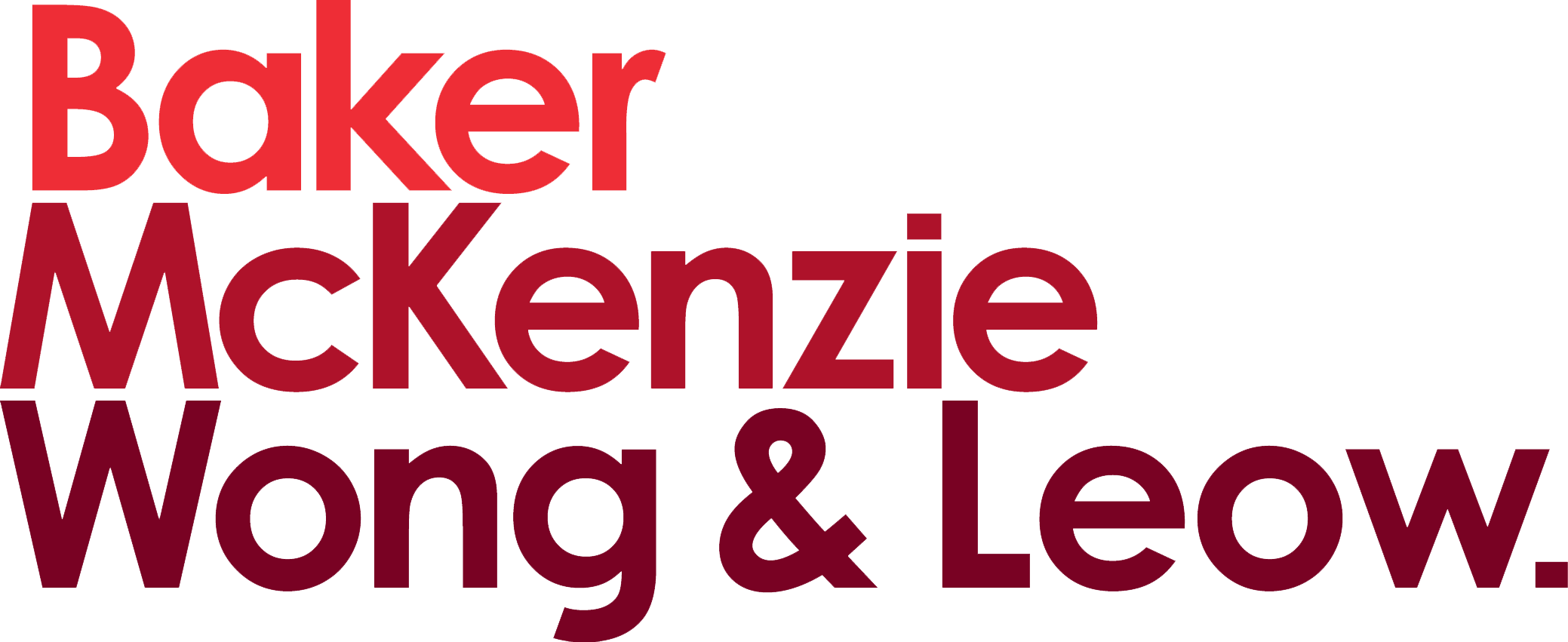
© 2024 Baker & McKenzie.Wong & Leow. All rights reserved. Baker & McKenzie.Wong & Leow is incorporated with limited liability and is a member firm of Baker & McKenzie International, a global law firm with member law firms around the world. In accordance with the common terminology used in professional service organizations, reference to a "principal" means a person who is a partner, or equivalent, in such a law firm. Similarly, reference to an "office" means an office of any such law firm. This may qualify as "Attorney Advertising" requiring notice in some jurisdictions. Prior results do not guarantee a similar outcome.